
Hey there. I'm James!
I am a builder based in San Francisco currently working on Redraft, an AI workspace that helps enterprise sellers research potential customers. Previously, I was the Founder of Alohop and a Product Manager at DataRobot.
I love creating simple products that solve complex problems. Having worked as a PM at DataRobot, where I helped deploy AI for large enterprises, and as a product engineer at Redraft, I've been fortunate to be deeply immersed in the engineering and go-to-market cycles required to deliver AI to enterprises and consumers.
Outside of work, I was the captain of Indonesia's U18 basketball national team and continue to perfect my jump shot.
My Work
Other Projects
My Links
My Work
Redraft.ai
Currently, I'm building Redraft to help enterprise sellers research and sell into big companies. Now with paying customers, it's the fastest way for sellers to figure out what to say and who to reach out to when targeting a new company.
As a Product Manager at DataRobot, I saw firsthand how much time account executives spent on company research. I built Redraft with feedback from 30+ sellers to tell them everything they need to know about a company in minutes.
Beyond solving this problem, my goal was to get engineering experience bringing a generative AI product from 0 to 1, where I had to build and orchestrate everything myself. After a few months, Redraft has become the single fastest way for an enterprise seller to research companies and is now in public beta.
"Your product is fantastic, especially the automatic contact identification - it's far superior to Sales Navigator and ZoomInfo. The tool is proving invaluable for channel partner meetings. Having deep account knowledge beforehand helps us better position our solution to their customers." - Paying customer from GPU provider with $100m+ funding.
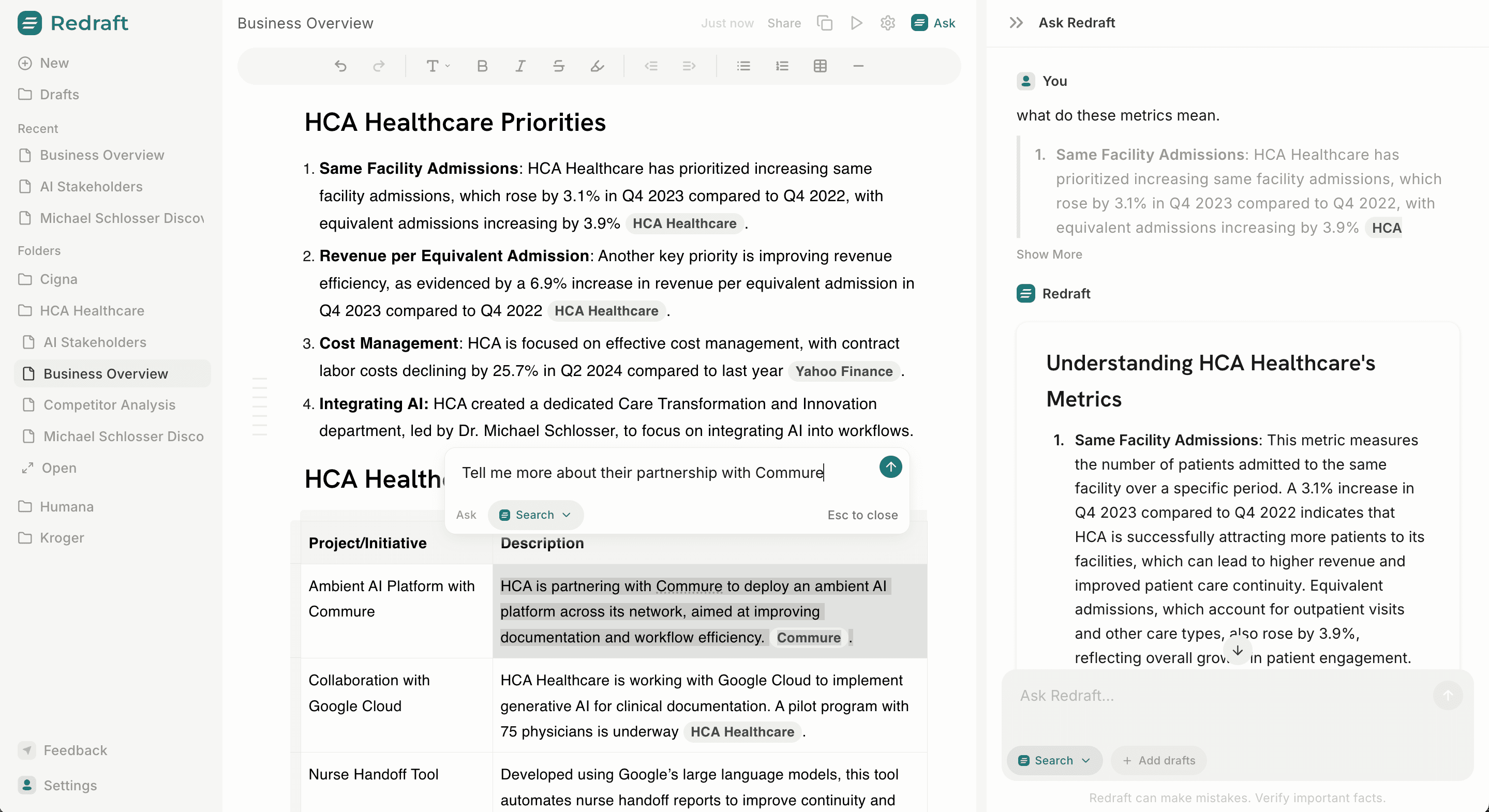

Alohop
Previously, I founded Alohop and raised $2.2M to build the OpenTable for in-person experiences. In 2021, as work went remote, our thesis was that more of the money typically spent on travel would shift to local experiences.
The problem was that local experiences—ranging from fencing classes to cooking workshops—were hard to find because they were scattered across generic platforms like Groupon and Eventbrite. We also saw an opportunity to expand the market by helping retail shops that didn't typically offer experiences to start doing so to drive foot traffic - for instance, helping a candle shop host candle-making workshops.
In short, our goal with Alohop was to bring together all the best local experiences in a city, personally curate them for quality, and create a seamless platform where people could book and review with confidence.
We launched in Boston and in 18 months generated $270,590 in GTV for local businesses and acquired 7,058 paying customers. As a founder, I not only designed the platform and led the engineering team but also did door-to-door sales to onboard each local business myself.
On supply, we pushed through the cold-start problem, manually building high-quality supply from scratch. On demand, we drove bookings through word-of-mouth, TikTok, and Instagram. On product, we integrated with all the major booking platforms, built manual scrapers for dozens of websites, and created a great online booking experience.
Unfortunately, our data showed that our marketplace wasn't high-frequency or high-ticket enough to achieve desirable unit economics. Unlike accommodations, where people spend large amounts per booking, or food delivery, where power users order daily, local experiences lacked both the transactional volume and repeatability required for a vibrant, scalable marketplace.
We explored different models, including helping individuals turn their skills into experiences to generate income and create novel supply. However, paired with the return to office, we ultimately didn't see local experiences as a viable space and decided to return capital to investors.
Personally, it was an incredible journey that taught me so much about building for consumers, launching a marketplace, and leading a company.
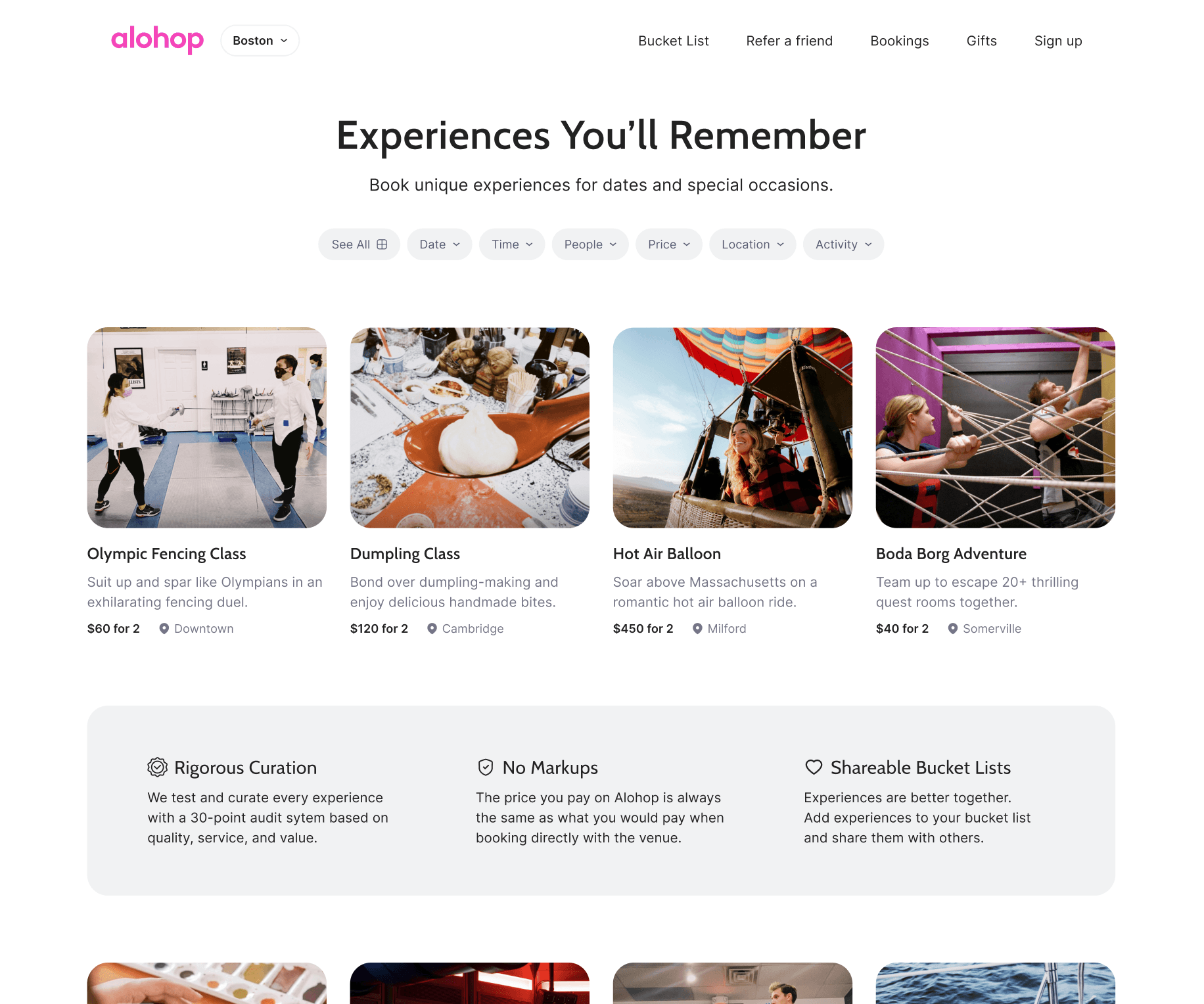

DataRobot
In two years at DataRobot, I drank straight from a firehose. I was lucky to land a role at the company during its period of hypergrowth, starting with its Solutions team. In my first week, I asked 20 sales and success reps a simple question: What are your biggest challenges? The answer was unanimous—since DataRobot was industry-agnostic, customizing relevant use cases for every new prospect was difficult.
That insight shaped a big part of my experience at the company. I started by building an internal tool that gave sellers and customer success teams complete transparency into every AI use case DataRobot had deployed. To do this, I manually reviewed thousands of use cases in Salesforce and identified the ones that reached production. I then interviewed 50+ data scientists who had worked on those accounts to understand every detail and enrich our knowledge base.
I was looking for patterns—signs of repeatability that sellers could leverage. This effort evolved into my first public-facing product, the AI Library, which I pitched to our CEO for support before leading a team to build it. In the meantime, I was heavily involved in major deals, helping customers design and deploy highly valuable applications in their businesses, primarily in healthcare and retail.
Thanks to my deep understanding of customer needs, I was promoted to Product Manager for the Federal team, where I helped design and launch AI products and solutions for our largest public sector customers. This was the most fulfilling period of my time at DataRobot, where we used machine learning to address some of the country's most critical problems, particularly those related to the pandemic.
Additionally, as a Product Manager, I helped develop DataRobot's first self-serve product, enabling new users to create machine learning models using public datasets related to common industry use cases. I also helped design and launch features that allowed customers to track their deployed models and measure business savings based on the value of each prediction.
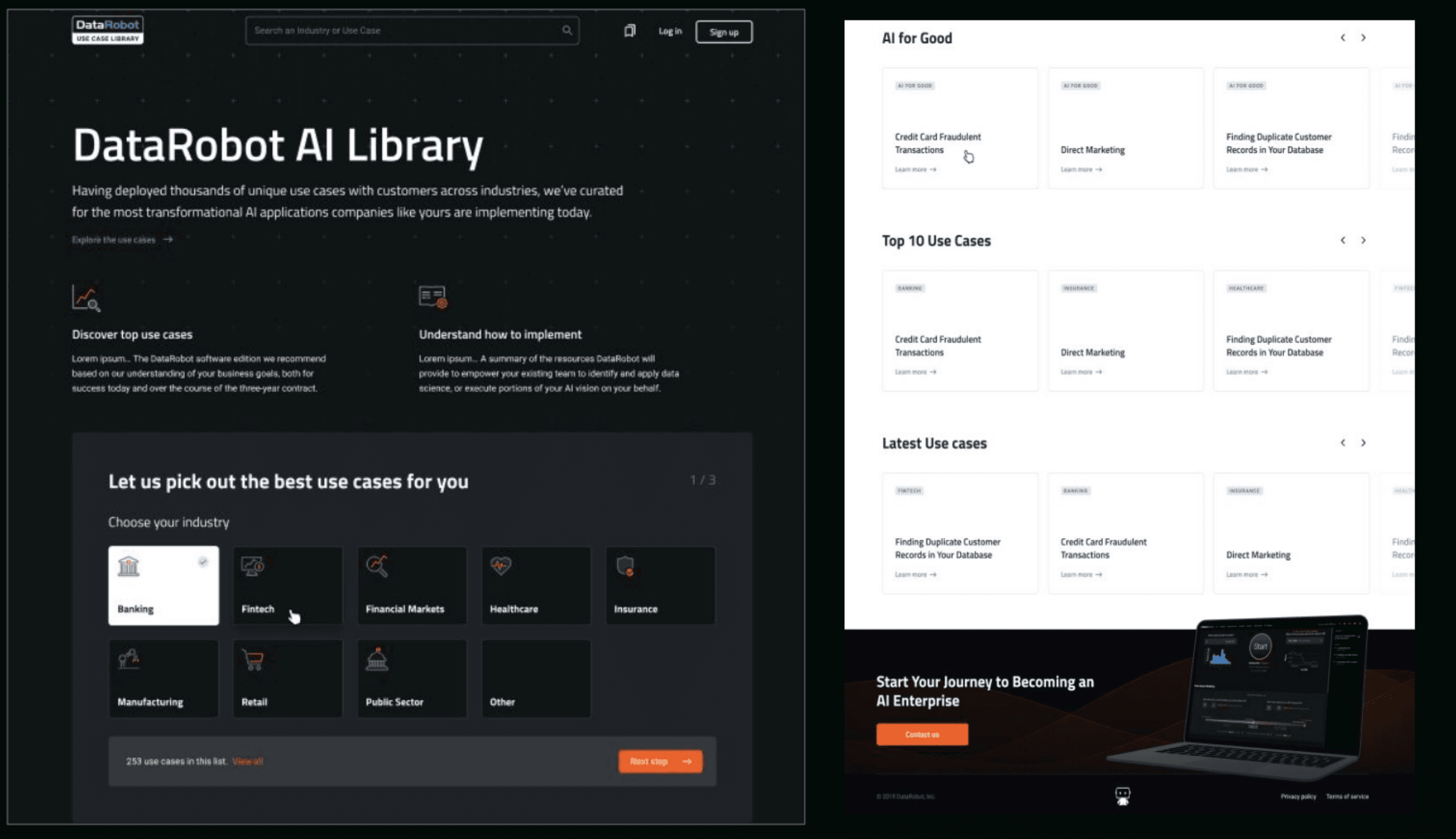
Other Projects
Outside of my main work, I let myself be guided by curiosity to work on projects that help me learn. My rule of thumb is to take these curiosities seriously - to obsess about them, talk to as many relevant people as I can, and build on my own to get deep technical experience.

Semua.ai
SemuaAI was ChatGPT for Indonesia. Using a test dataset created by Indonesian researchers, I found that GPT-4 only scored 56.68% on Indonesia's college entrance exams. While there is plenty of Indonesian content online, most rich Indonesian text—especially legal and medical knowledge—still exists primarily in physical textbooks that haven't been digitized.
I relocated to Indonesia for eight months and partnered with a co-founder to build the product. I led our MVP development, pitched to local companies, and oversaw our data collection efforts. My co-founder, a data engineer, fine-tuned Llama models using transcribed content from textbooks we digitized. We gained valuable experience fine-tuning language models, but we quickly realized that scaling required far more resources and formal partnerships with local publishers.
From the beginning, we knew Indonesia's consumer market wouldn't support a subscription-based model due to limited purchasing power. Our goal was to validate the local enterprise market to gauge whether it could support a venture-scale business.
However, we learned that the enterprise market wasn't large enough to justify the investment. We found that, unlike China and India—where knowledge-based economies create strong demand for LLMs—Indonesia's economy is driven by natural resources and manufacturing, leaving only a few hundred potential enterprise customers across banking, retail, legal, and consulting.
While we decided that launching an Indonesian-focused AI research lab wasn't viable, the experience that gave me a deeper understanding of model fine-tuning and localization.
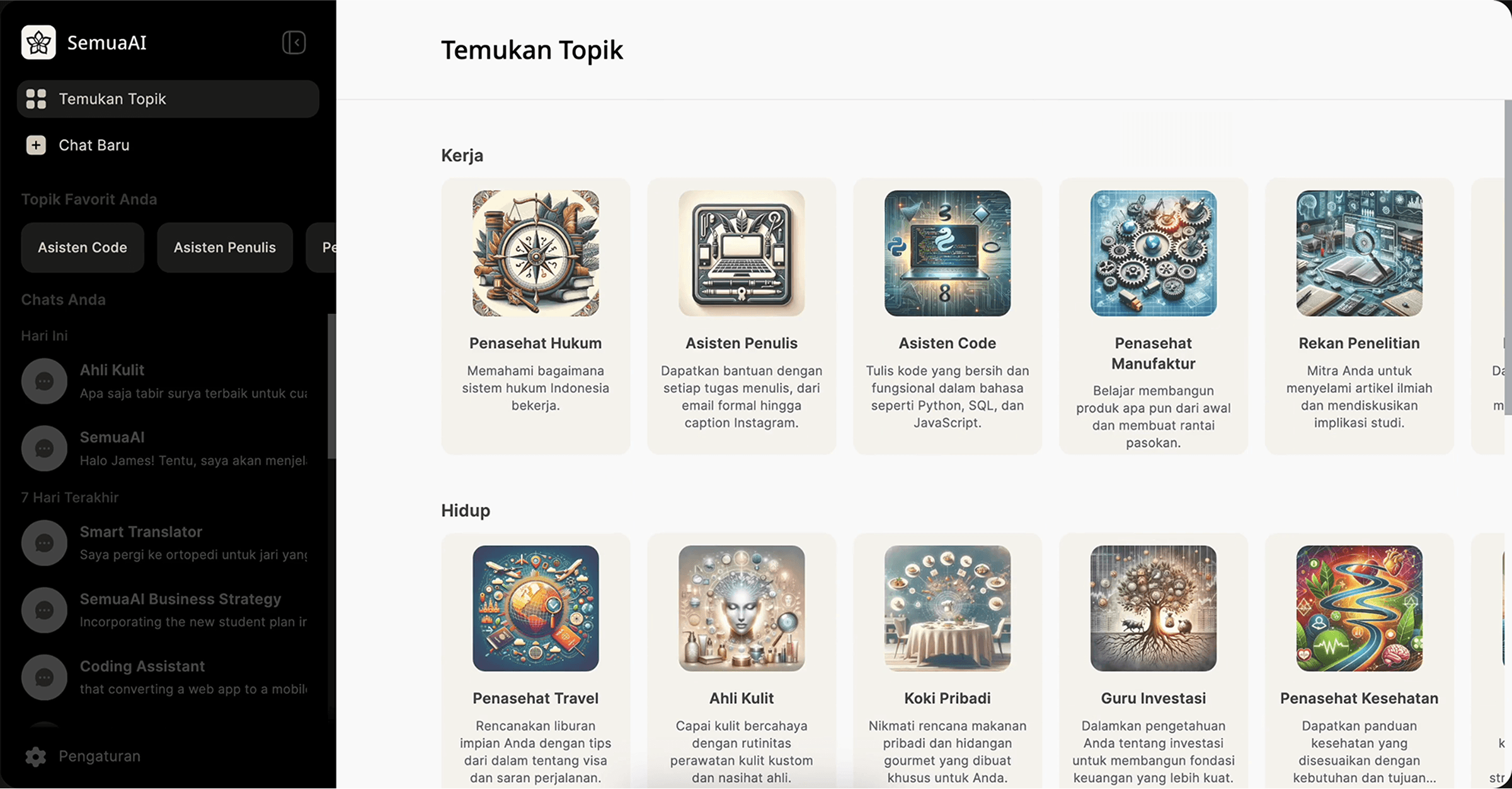

DreamSquad
DreamSquad was the first generative AI product I built. This was pre-GPT Store when ChatGPT could only handle a single prompt at a time. The idea was to create Character.ai for Work—a workspace where each AI character specialized in a specific domain like Product, Engineering, or Marketing and was integrated with different tools to act as a real teammate.
I designed and built DreamSquad myself, learning how to give AI characters infinite memory with RAG to overcome GPT-4's limited context window. I also developed workflows that allowed different AI characters to communicate, ensuring that insights weren't siloed and that each "coworker" could reference relevant context from others. This structure mirrored how people naturally switch between different experts in a team.
When OpenAI launched the GPT Store, it became clear that DreamSquad lacked the depth needed to stand out. Instead of pursuing a broad AI workspace, I chose to focus on a single high-value vertical. That decision led me to build SemuaAI to explore ChatGPT for Indonesia and, eventually Redraft, where I'm now focused on helping enterprise sellers.
DreamSquad was an important stepping stone—it gave me hands-on experience designing AI systems, integrating RAG for persistent memory, and imagining how users can interact with AI in a collaborative setting.
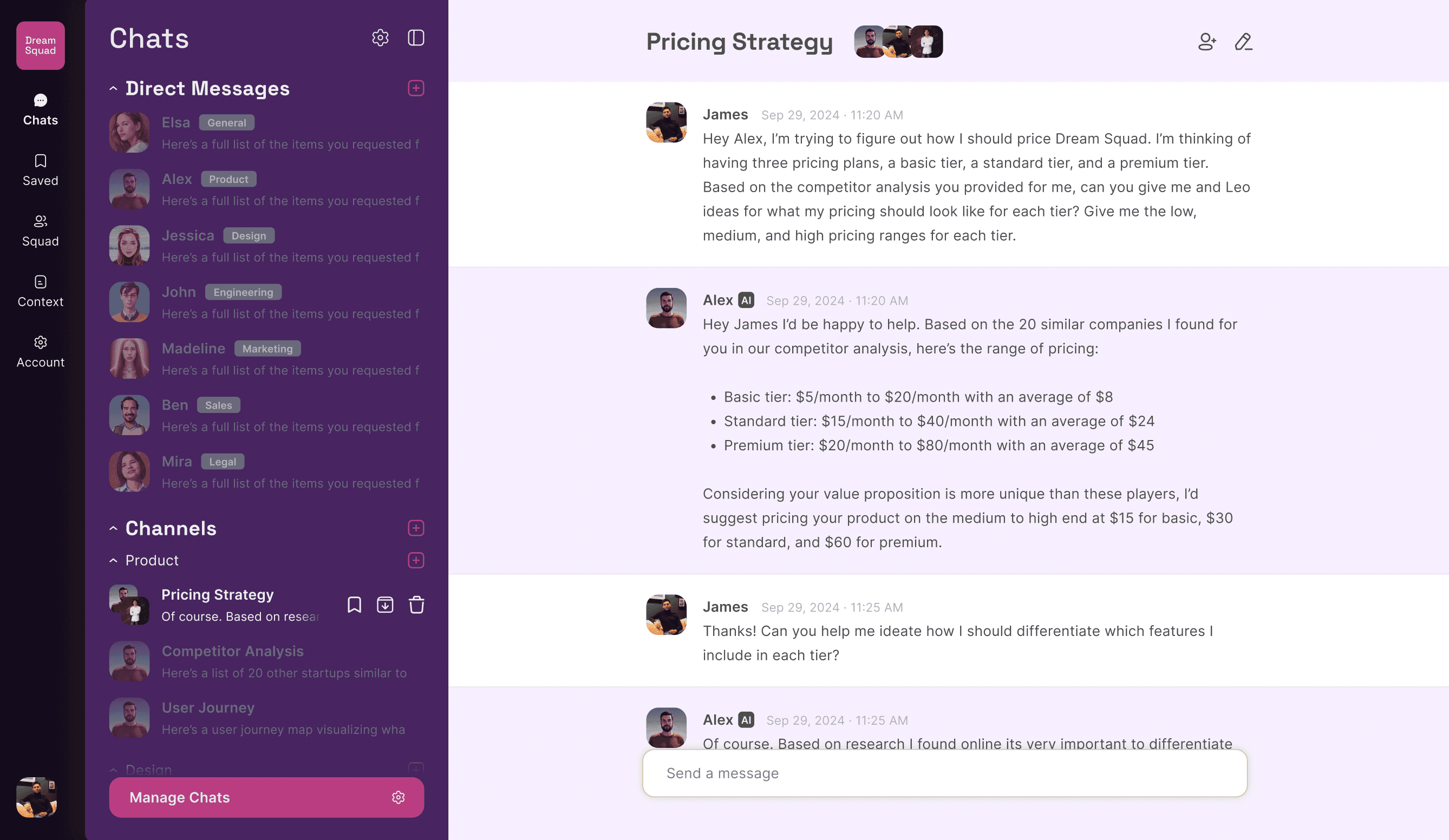

Traveloka
As Southeast Asia's largest online travel agency, Traveloka worked with thousands of accommodation providers. To uncover new product opportunities, I led a team of five to interview 1,000 accommodation providers across the region to learn their pain points.
I designed and led our outreach process. We identified the most prominent accommodations in Indonesia and called each of their General Managers. I built a mini call center operation—hiring five people, writing scripts, creating rebuttals, and managing the team. I personally conducted many of the calls myself.
Looking back, this was my first experience designing a go-to-market motion from scratch. Not only did I develop the system for gathering insights, but I also had to "sell" these accommodation providers on why they should share their feedback with us. That hands-on approach shaped how I think about product discovery today.
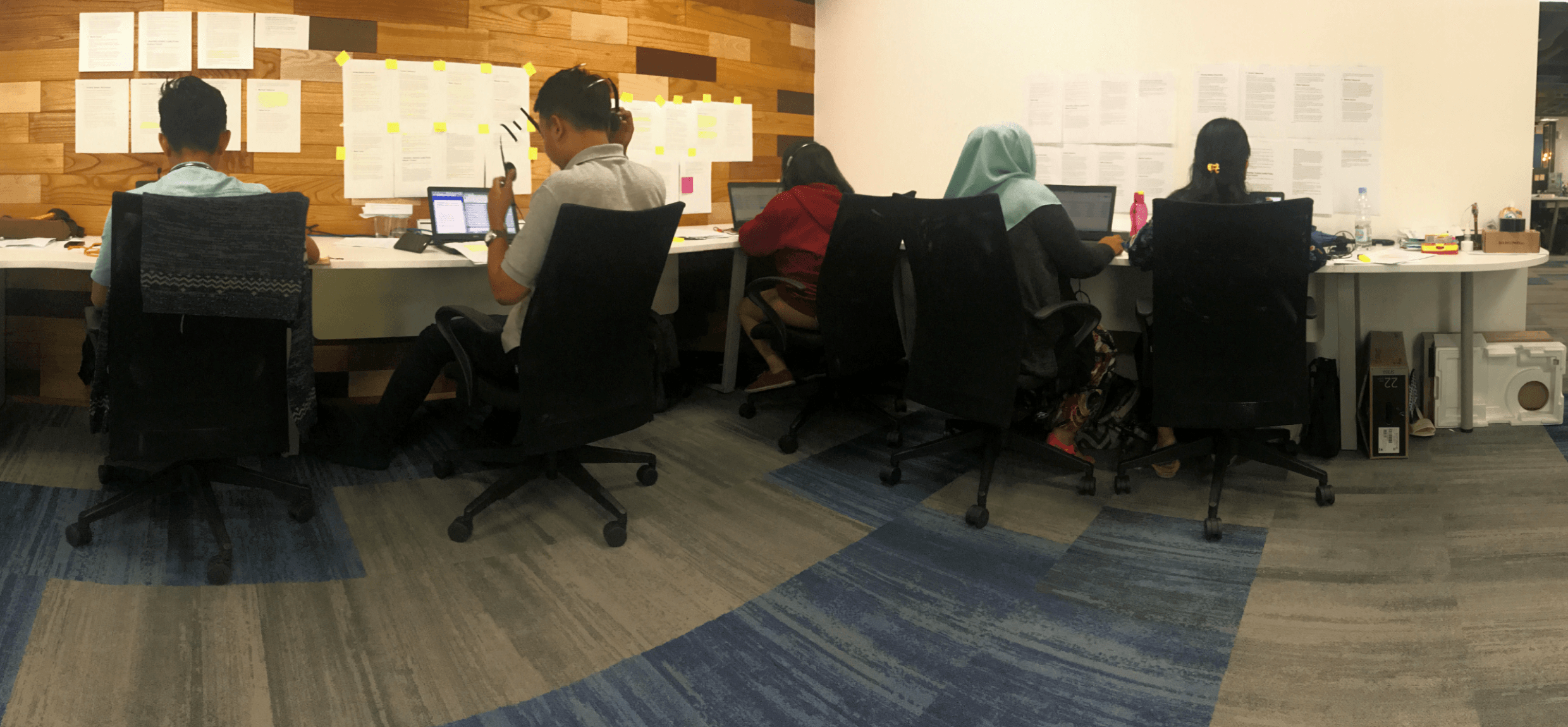

Orange Papaya
The first product I ever built wasn't software—it was a notebook. But not just any notebook. It was the world's most ethical notebook.
In college, I saw that many underprivileged women in Indonesia were unable to earn an income because they had to stay home to care for young children. At the same time, their communities were overrun with waste. Meanwhile, in the U.S., the demand for eco-friendly products was booming.
I partnered with a local nonprofit to create a solution. We helped these women generate income by crafting handmade notebooks using recycled newspapers. They made the covers, while I sourced local suppliers to produce the inner pages from reused paper. I designed the entire supply chain and manufacturing process, before selling the notebooks through a successful Kickstarter campaign and retail stores in Boston.
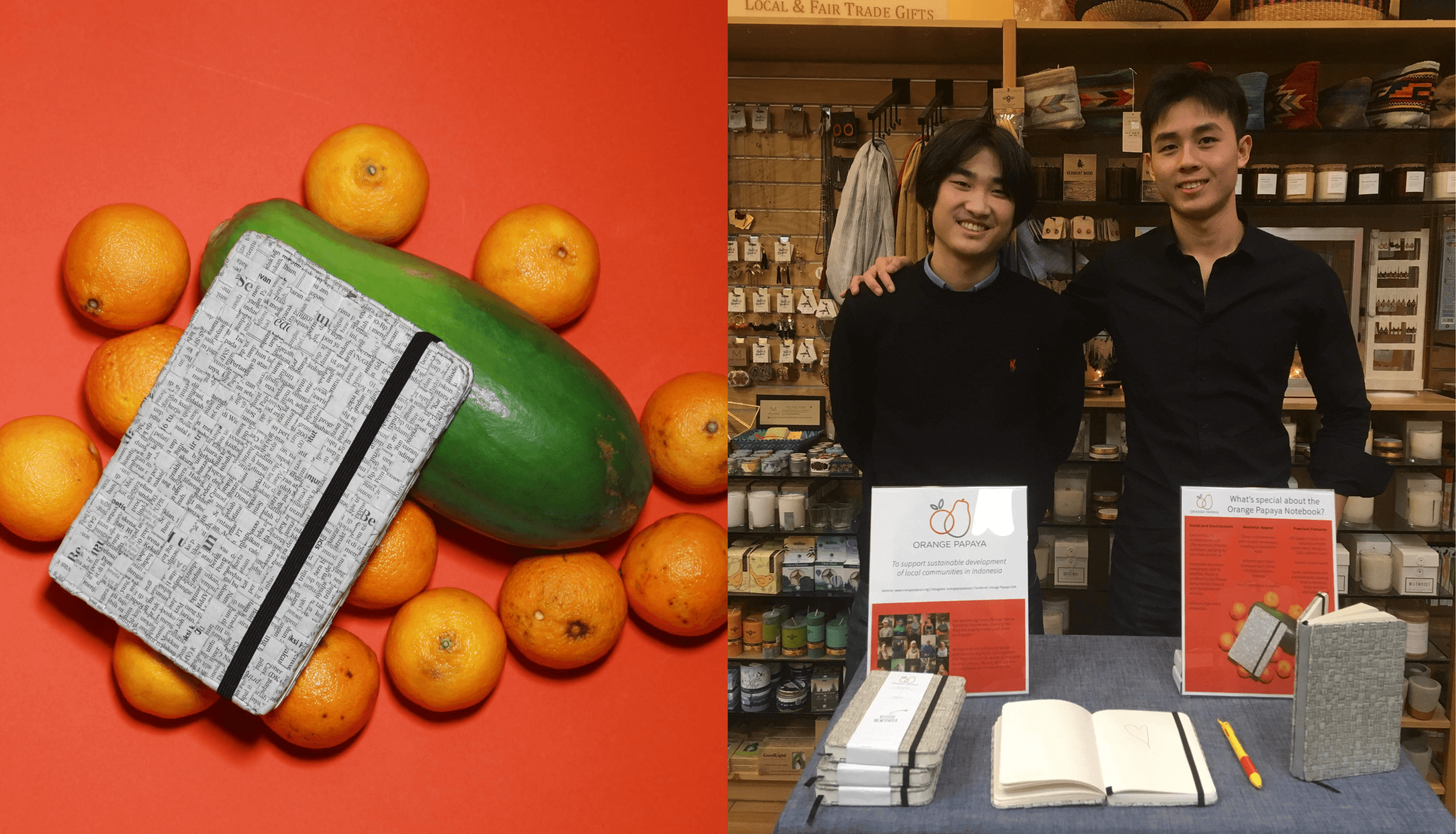